Introduction
Palabuhanratu Bay and its surroundings in West Java Province, Indonesia, are promising fishing grounds, particularly for pelagic fish species. The spatial distribution of sea surface temperature affects the temporal distribution of fishing grounds in Palabuhanratu waters (Ghufron et al., 2019). This demonstrates that variations in the migration activity, distribution, and abundance of pelagic fish in water are influenced by oceanographic parameters (Apriansyah et al., 2023; Jurado-Ruzafa et al., 2019). The research results by Nurdin et al. (2018) proved that the chlorophyll-a concentration is another important factor influencing the distribution of fish resource abundance in a water body. Apriansyah et al. (2023) also emphasized that the chlorophyll-a concentration can predict fish dispersal habitats. Therefore, oceanographic data are critical for investigating the pelagic fishing grounds in Palabuhanratu.
However, accurate predictions of fishing grounds remains a significant challenge. The effectiveness of fishing operations is closely tied to the precision of these predictions. Fishers sometimes rely on model and remote sensing technologies to identify productive fishing grounds (Han et al., 2023). However, the adoption of these methods varies, with some fishers using them regularly while others rely on traditional methods. According to Nurani et al. (2021), identifying the presence of fish in water is crucial determining the optimal location and timing of fishing. In addition, knowledge of fish-aggregating devices (FADs) can significanly enhance the efficiency of fishing operations byreducing risks, time, and costs (Raju et al., 2022).
Modeling technique, such as generalized additive modeling (GAM) and the maximum entropy model (MaxEnt), are commonly employed to predict potential fishing grounds based on ecological suitability (Mazurkiewicz, 2015). GAM and the MaxEnt are two modeling methodologies often used to determine possible fishing grounds based on ecological appropriateness. GAM is frequently used to assess fish habitats, and support management and conservation decisions by incorporating environmental variables (Coletto et al., 2018; Salazar et al., 2021). On the other hand, the MaxEnt predicts fishing spots by integrating multiple environmental factors, offering a straightforward and effective approach to data processing (Philips et al., and Dudik, 2009; Holder et al., 2020).
Pratama et al. (2022) successfully apllied the MaxEnt method to model the suitability of pelagic fish habitats in Palabuhanratu Bay and its surroundings. This model utilized oceanographic parameter such as chlorophyll-a levels, sea surface temperature, sea surface salinity (SSS), currents and bathymetry. The data were spatially mapped to generate a habitat suitability index (HSI). The HSI reflects the likelihood of pelagic fish presencein specific water bodies. The higher HSI values indicating greater suitability for pelagic fish habitats. This spatial map of HSI can be a valuable tool for predicting potential fishing grounds in Palabuhanratu Bay and its surroundings.
To ensure the practical application of this model, it is essential to evaluate and refine the implementation strategy. Interpretive structural modeling (ISM) offers a robust approach for developing a strategy by analyzing and prioritizing key elements that influence the successful implementation of the model. ISM simplifies complex systems by organizing expert opinions into a hierarchical structure, making it easier to identify and address the critical factors (Ahmad & Qahmash, 2021; Li et al., 2019). The ISM is a strategic modeling technique that organizes and summarizes experts' opinions on the hierarchical structure of system elements. The ISM effectively analyzes the impact of one variable on (Gardas et al., 2017). A key outcome is its ability to identify key elements that are essential for successful implementation. By prioritizing these elements, ISM helps formulate effective strategies. This technique simplifies complex systems, aids in structural analysis, and provides up-to-date, quantitative insights, making it widely adopted by researchers and (Bag & Anand, 2015; Luthra et al., 2011).
This study aims to develop a strategy for implementing a predictive model for identifying potential pelagic fishing zones in Palabuhanratu Bay and its surroundings. This research is essential for implementing strategies this model in the field. The findings of this study may help achieve sustainable development goals (SDGs) by reducing travel time to fishing grounds, finding new fishing spots, consuming less fuel, and increasing profitability.
Materials and Methods
This study was conducted in Palabuhanratu Bay and its surroundings, Sukabumi Regency, West Java Province, Indonesia, at 6.50°S–7.20°S and 106.00°E–106.40°E, as shown in Fig. 1.
The implementation strategy of the pelagic fish habitat suitability model in Palabuhanratu was formulated using ISM. ISM is a methodology that helps to identify and structure the relationships among elements within a complex system, thereby facilitating the development of an effective implementation strategy (Maulina et al., 2021; Nurani et al., 2011; Pfohl et al., 2011). In this study, ISM was employed to analyze the system elements, drawing on the experience and knowledge of experts to establish directional relationship, which were interpreted using an interaction matrix (Li et al., 2019).
The ISM method was applied to develop a suitable implementation strategy for disseminating the pelagic fish habitat suitability model effectively to target users. The stages of formulating this strategy, as outlined by Li et al. (2019) and Saxena et al. (1992), are illustrate in Fig. 2. The study’s outcome have the potential to reduce the time required to reach fishing grounds, identify new fishing locations, and lower fuel consumption, which aligns with the SDGs.
The ISM procedure systematically ranked the elements influencing the successful implementation of the pelagic fish habitat suitability model in Palabuhanratu and its surroundings. A purposive sampling technique was employed to select respondents for the study. Data collection was conducted through in-depth interviews with experts, considering two key criteria: 1) the availability and willingness to participate, and 2) the expert’s position, experience, and credibility in fisheries resource utilization.
Six respondents participated in the research, including representatives from the Marine and Fisheries Agency (MFA) of West Java Province, the MFA of Sukabumi Regency, the Head of the Palabuhanratu Fishing Port, Directorate General of Marine and Fisheries Resources Surveillance (a government agency under the management of the Ministry of Marine Affairs and Fisheries of Indonesia), and representatives from troll line and longline fisher groups. According to Hora (2004), the appropriate number of expert respondents ranges from 3 to 7. Before conducting the interviews, detailed explanations of the research background, the problems being address, research objectives, and modelling results were provided to the respondents. The prototype of the system, which is an Android application displaying the pelagic fish habitat suitability model results as a map of potential fishing area, was also presented. This application allows fishers to access information on potential fishing zones in Palabuhanratu Bay and its surrounding areas.
The initial step in formulating a strategy using ISM is to identify the elements of the system and establish the contextual relationships between them. The elements and their contextual relationships may vary depending on the specific location and scale of the study. In this research, the selected elements were based on the work of Li et al. (2019) and Saxena et al. (1992), which include objectives, major obstacles, required activities, institutions/parties involved, and sectors of society affected.
The first element, the objective, outline of the goal of implementing the prediction model results. To achieve this goal, various activities are necessary, which are categories under the element of required activities. Each activity involves specific institutions/parties that play a crucial role in the successful implementation of the model, addressing the key constraints that might impede the achievement of the objective. Successfully achieving the objective is expected to have positively impact on multiple parties, which are represented by the sectors of society affected.
Once the elements are determined, the next step is to identify the sub-elements for each element. Sub-elements are defined through a combination of literature studies, direct observations, and expert opinions. The identification elements and sub-elements relevant to the model implementation are presented in Table 1.
The second phase involved the creation of a structural self-interaction matrix (SSIM) based on the results of the questionnaires and expert interviews. The matrix was compiled using the symbols V, A, X, and O as described by Sinaga et al. (2019) and Zaidi et al. (2021). The third step was the preparation of the reachability matrix (RM) by converting the symbols in the SSIM into numerical values, either 1 or 0, according the following conditions (Sushil, 2018):
After obtaining the RM value, the next step involved correcting these values according to transitivity rules to produce the final RM value (Bag & Anand, 2015; Sushil, 2018; Zaidi et al., 2021). Once the final RM values were obtained, the consistency of the experts agreement was tested using the following formula:
with n: number of sub-element
Y: number of modifications following transitivity rules
If the test results indicated inconsistencies in the conceptual relationships, it suggested possible errors in establishing the relationships between the elements. To minimize these errors, semi-structured interviews were conducting to adjust any inaccurate relationships between elements (Li et al., 2019).
The subsequent step was to construct a structural diagram of the key elements and the driver power-dependence matrix. In this structural diagram, key elements can be identified based on the level of each sub-element. The sub-element with the highest level within an element is considered the key element of that particular element. The driver power-dependence matrix is then used to determine the strength and relationships of each sub-element. The matrix divided of four sectors: autonomous, dependent, linkage, and independent (Nurani et al., 2011; Saxena et al., 1992; Zaidi et al., 2021), as shown in Fig. 3.
Results
This study identified five key elements, as detailed in Table 1. Fig. 4A–4E illustrates the driver power-dependence matrix for each element, while Fig. 5A–5E depicts the structural model for each element. A detailed explanation of each element is provided below.
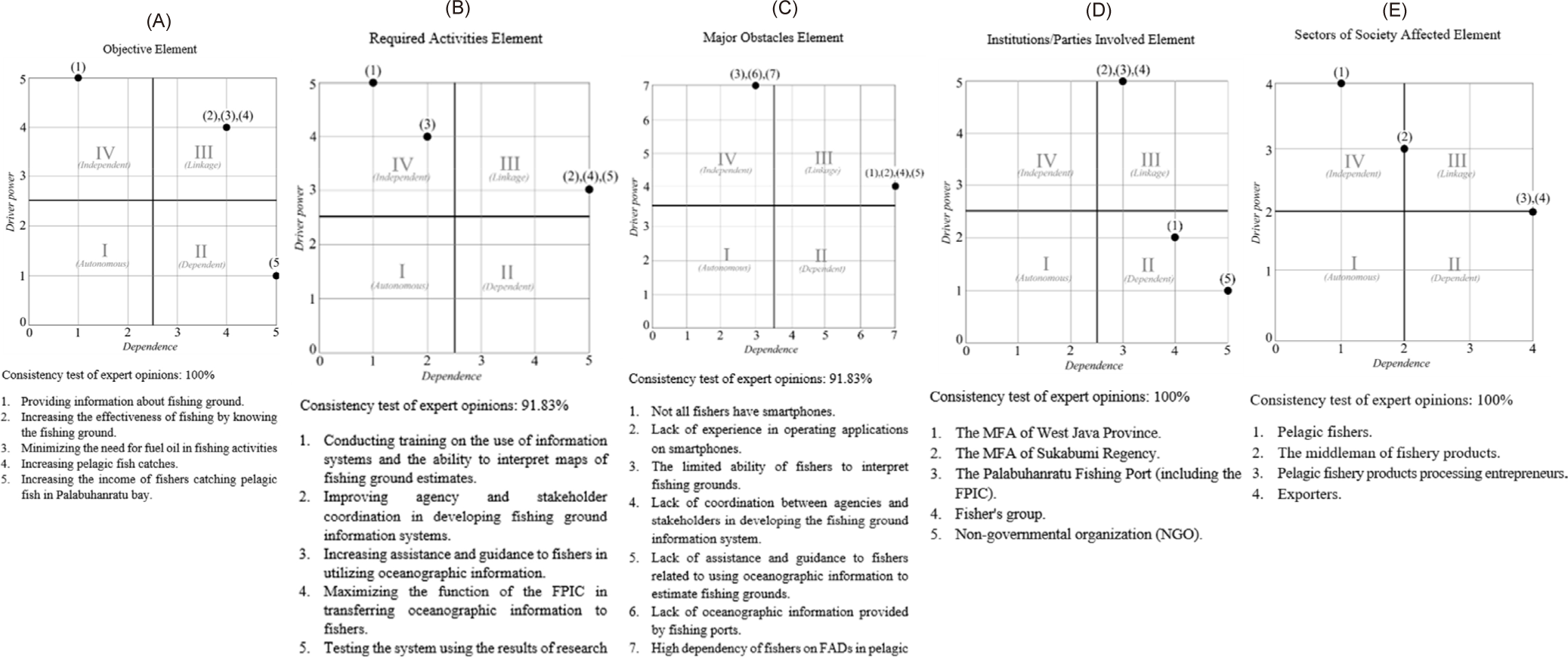
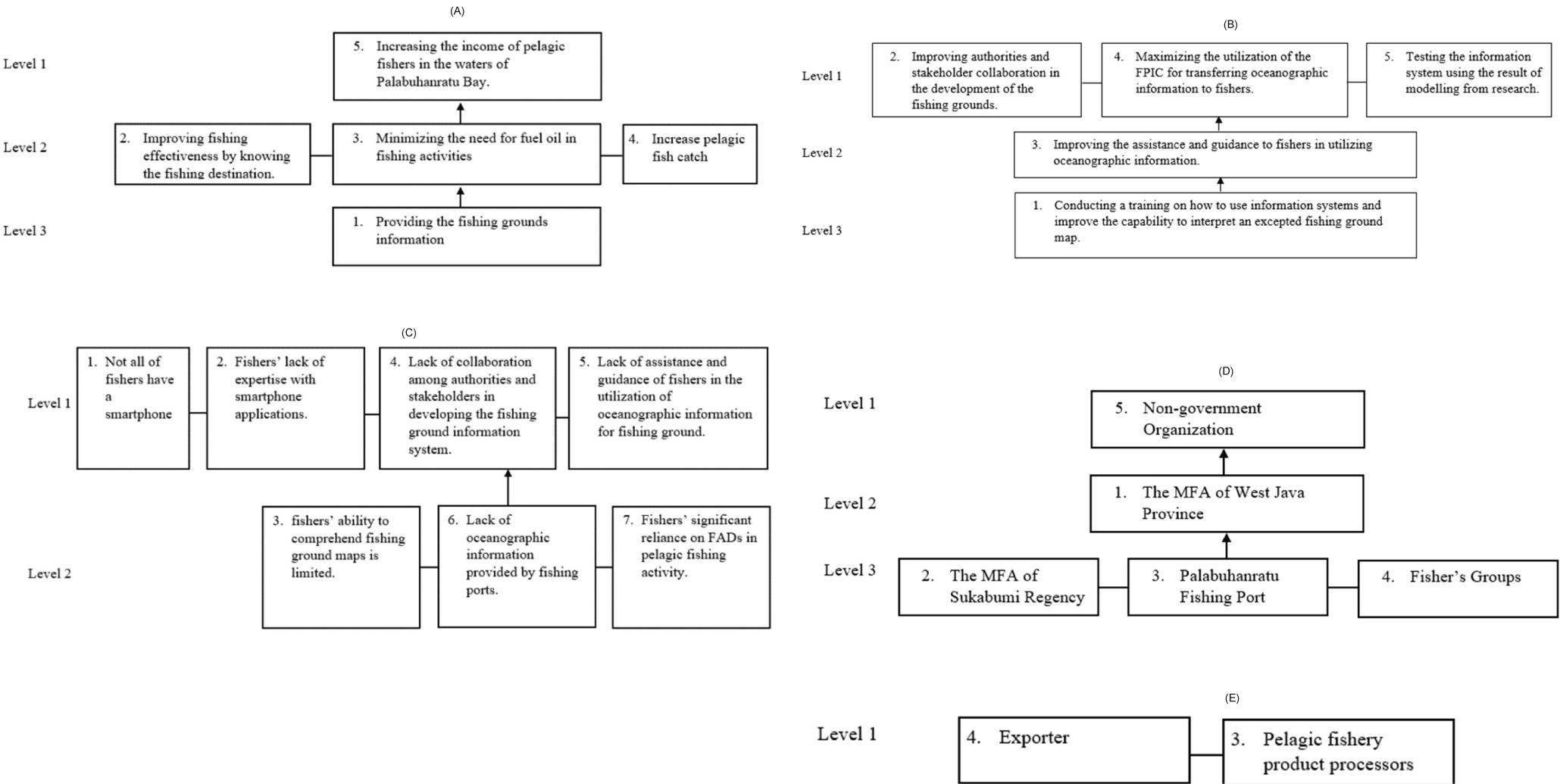
The objective element comprises five sub-elements, as listed in Table 1. The consistency test of expert opinions for this element yielded a result of 100%, indicating high consistency among respondents. Fig. 4A presents the driver power-dependence matrix for the sub-elements. Sub-elements (2), (3), and (4) are classified as sector III, suggesting they possess both strong driving force and strong inter-element interactions. Sub-element (1) is located in sector IV, indicating a strong driving force but a relatively weak interaction with other sub-elements. Sub-element (5) is positioned in sector II, implying a weak driving force but a reasonably strong interaction with other sub-elements. The structural model diagram for the objective element, shown in Fig. 5A, consists of three levels: Level 1 includes of sub-elements (5); Level 2 comprises of sub-elements (2), (3), and (4); and Level 3, identified as the key element, includes of sub-element (1).
In addition, the activity element in this research consisted of five sub-elements, as listed in Table 1. The consistency test for expert opinion regarding this elements yielded a value of 91.83%, demonstrating consistent views among respondents. The driver power-dependence matrix for the sub-elements is shown in Fig. 4B. Sub-elements (2), (4), and (5) are located into sector III, indicating strong driving force and robust relationships between elements with other sub-elements. Sub-elements (1) and (3) are in sector IV, meaning they have a strong driving force, but a relatively weak relationship with other sub-elements. The structural model diagram for the activity element, as shown in Fig. 5B consists of three levels: Level 1 indicates of sub-elements (2), (4), and (5), Level 2 includes sub-elements (3), and Level 3, identified as the key elements, includes sub-elements (1).
The constraint element consists of seven sub-elements, as detailed in Table 1. The expert opinion consistency test for this element also yielded a value of 91.83%, reflecting consistent respondent opinions. Fig. 4C presents the driver power-dependence matrix for the sub-elements. Sub-elements (1), (2), (4), and (5) fall into sector III, indicating strong driving forces and significant relationships between elements. Sub-elements (3), (6), and (7) are in sector IV, suggesting they have strong driving force but a relatively weak relationship with other sub-elements. The structural model of the constraint element is shown in Fig. 5C, consists of two levels: Level 1 consists of sub-elements (1), (2), (4), and (5); and Level 2, identified as the key elements, includes sub-elements (3), (6), and (7).
The institutional elements involved five sub-elements, as listed in Table 1. The consistency test for expert opinion yielded a result of 100%, indicating high consistency among respondents. The driver power-dependence matrix are shown in Fig. 4D. Sub-elements (2), (3), and (4) are positioned into sector III, indicating strong driving force and robust relationship with other elements. Meanwhile, sub-elements (1) and (5) fall into sector II, indicating weaker driving force but stronger interactions between sub-elements. The structural model diagram for the institutions element, illustrated in Fig. 5D, consists of three levels: Level 1 consists of sub-elements (5); Level 2 includes of sub-elements (1); and Level 3, identified as the key elements, includes sub-elements (2), (3), and (4).
The affected community consisted of four sub-elements, as detailed in Table 1. The expert opinion consistency test yielded a score of 100%, demonstrating highly consistent viewpoints among respondents. Fig. 4E shows the plot of the sub-elements in the driver power-dependence matrix. Sub-elements (2), (3), and (4) are positioned into sector III, indicating strong driving forces and relationships between elements. However, sub-element (2) has slightly different characteristics, showing lower interaction with other sub-elements but a higher driving force compared to sub-elements (3) and (4). Sub-element (1) is located in Sector IV, suggesting it has the strongest driving force but relatively weak interactions with other sub-elements. Sub-element (1) is located in Sector IV, suggesting it has the strongest driving force but relatively weak interactions with other sub-elements. The structural model diagram for the affected community elements is shown in Fig. 5E, consists of three levels: Level 1 consists of sub-elements (3) and (4); Level 2 consists of sub-elements (2); and Level 3, identified as the key element consists of sub-elements (1). The key elements of each element are listed in Table 2.
Discussion
The key elements generated in formulating implementation strategies through ISM are critical factors that affect the success of implementation (Maulina et al., 2021; Rizal et al., 2016). A driver power-dependence matrix (Fig. 3) and model structure diagram (Fig. 4) were developed to analyse the target element. According to the driver power-dependence matrix, sub-element (1) of the objective element, which provides information on fishing grounds, is in sector IV, indicating that it has high driving power and low system dependence. This sub-element has a high driving force because it strongly influences or stimulates the realization of other sub-elements. However, sub-element (1) can be realized independently without needing support from other sub-elements. Sub-element (1) is a key element; therefore, achieving the goals associated with this sub-element is crucial for accomplishing the objectives of other sub-elements.
The sub-elements in sector III required careful consideration and prioritization for the program’s success (Palobo & Yuliadi, 2019). This is due to the fact that sector III has significant relationships and driving forces with other sub-elements, so failure to implement sub-elements in this sector will lead to the failure of other sub-elements. Therefore, if these sub-elements are not given special attention, they may become obstacles to the overall implementation of the program. (Nurani, 2010). One of the key goals that system actors, such as fishing ports and maritime and fishery agencies, must achieve is providing accurate information on fishing grounds. According to Apriliani & Nugroho (2016), fishers seek information on the prediction of fishing grounds based on this application. The availability of such information, based on oceanic modeling results, can assist fishers in reducing fuel costs and increasing fishing effectiveness (Raju et al., 2022). Therefore, specific activities are activities are necessary to achieve these goals.
Activities with a strong driving force but low dependency (sector IV) on the system are found in sub-element 3 on required activities element, which involved conducting training on the use of information systems and the ability to interpret maps of suspected fishing grounds by fishers, and sub-element 1, which focusing on increasing assistance and guidance to fishers in utilizing oceanographic information. Successfully implementing these activities will enhance fishers’ ability to use oceanographic data and predicted fishing ground maps. This, in turn, will facilitate other activities, such as optimizing the FPIC role in transferring oceanographic data and testing the predicted fishing grounds system, allowing these to be fully realized and effectively utilized by fishers. Sub-element 3 is identified as a key element in the required activities, and thus, prioritizing these activities is essential for achieving the program's implementation objectives (Maulina et al., 2021). Training and mentoring on using oceanographic information and fishing ground maps are critical efforts to optimize fish resource availability and improve fishing effectiveness, ensuring the success and sustainability of fishing companies (Geronimo et al., 2018; Previero & Gasalla, 2018).
Implementing the model is inherently linked to addressing existing constraints. These constraints, anticipated throughout the implementation process, were identified based on expert conversations, field observations, and literature review. Constraints with a strong driving force and low dependency (sector IV) were found in the constraint sub-elements of the limited ability of fishers to interpret fishing ground maps (sub-element 3), lack of oceanographic information provided by fishing ports (sub-element 6), and high dependency of fishers on FADs in pelagic fishing activities (sub-element 7). As key elements in the major obstacles category, sub-elements 3, 6, and 7 must be prioritized for resolution to achieve the model's implementation objectives one approach to overcome these constraints is to successfully execute the required activities. For sub-element 3, the constraints can be mitigated through effective training programs focused on utilizing information systems and improving the ability of fishers to interpret predicted fishing ground maps. For sub-elements 6 and 7, constraints can be reduced by optimizing the FPIC in transferring oceanographic data and predicted fishing sites. The success of this required activities is directly tied to minimize the constraint elements. Completing the necessary actions and achieving the set goals will help reduce limitations, which is critical for the effective development of a model that fishers can use optimally.
The involvement of numerous parties is critical for the model’s successful implementation. Several key parties have been identified as playing essential roles. The implementation should involve the MFA of the Sukabumi Regency, the Palabuhanratu fishing port, and fisher groups (troll lines and longline fishers) as key elements within the affected sectors of society. The MFA of the Sukabumi Regency and Palabuhanratu fishing ports could coordinate the development of a prototype of the designed fishing ground information system, with one piece of information comes from the fish habitat suitability model. Coordination among sectors involved in a program is vital, as weak coordination can hinder the development of the fishery sector. Fisher organizations, which directly benefit from the modeling results, should work to enhance their ability to utilize oceanographic data and anticipate fishing grounds. Fisher groups can play a significant role in accelerating the development of information systems for the Palabuhanratu fishing ports.
The successful implementation of the model will have broad implication for numerous parties. The identification of those affected by the model’s success has been broken down into affected elements of society. According to the structural diagram of the model and the driver power-dependence matrix, pelagic fishers were identified as the key elements. Pelagic fishers have a strong driving force and minimal reliance on the program's performance. If they can effectively utilize the information system developed by the Palabuhanratu fishing port through the designed model or prototype, fishing effectiveness is expected to improve, leading to reduced fuel consumption.
All five essential components (goals, necessary tasks, primary program limitations, participating institutions, and impacted communities) were included when the model was successfully implemented. The primary actors to be considered when implementing this approach are fishers, whose primary need is to be informed of fishing areas. To ensure that important actions are completed successfully and to overcome the primary challenges in implementing this model, major institutions must perform their roles to the highest standard.
Conclusion
These key components require further consideration in strategies for effective model implementation. The main goal of pelagic fish habitat suitability modeling was implemented in Palabuhanratu Bay and its surroundings was to train fishers in using information systems and interpreting fishing-ground maps. Successful implementation depends on the strategic coordination and collaboration among the MFA of Sukabumi Regency, Palabuhanratu Fishing Port, and fisher groups. These institutions need to work together to address the major challenges: fishers’ limited ability to interpret fishing ground maps, the lack of oceanographic information at fishing ports, and the high dependence of fishers’ on FADs.